Proprietary Data from Data Partnerships: To further enhance the capabilities of o1 and o1-mini, we formed partnerships to access high-value non-public datasets. These proprietary data sources include paywalled content, specialized archives, and other domain-speci昀椀c datasets that provide deeper insights into industry-speci昀椀c knowledge and use cases. Data Filtering and Re昀椀nement: Our data processing pipeline includes rigorous 昀椀ltering to maintain data quality and mitigate potential risks. We use advanced data 昀椀ltering processes to reduce personal information from training data. We also employ a combination of our Moderation API and safety classi昀椀ers to prevent the use of harmful or sensitive content, including explicit materials such as CSAM. 3 Observed safety challenges and evaluations In addition to advancing language model capabilities, the o1 family’s ability to reason in context provides new opportunities for improving the safety of the model. The o1 models are our most robust models to date, achieving substantial improvements on our hardest jailbreak evaluations. They are also more aligned to the OpenAI policy, reaching state-of-the-art performance on our hardest internal benchmarks for evaluating adherence to our content guidelines. The o1 model family represents a transition from fast, intuitive thinking to now also using slower, more deliberate reasoning. While we 昀椀nd it exciting that reasoning can signi昀椀cantly improve the enforcement of our safety policies, we are mindful that these new capabilities could form the basis for dangerous applications. In this section, we outline the safety evaluations we conducted on this model, spanning harmfulness, jailbreak robustness, hallucinations, and bias evaluations. We then investigate risks involving the chain of thought itself, and describe our ongoing research on chain of thought deception monitoring. Finally, we detail the results of our external red teaming campaign. Additionally, as part of our continued e昀昀ort to partner with external experts, a set of pre- deployment evaluations were conducted on a version of the o1 model by the U.S. AI Safety Institute (US AISI) and the UK Safety Institute (UK AISI), not included in this report. Wecontinuously re昀椀ne and improve our models as part of our commitment to iterative deployment. The evaluations described in this System Card pertain to the full family of o1 models, and exact performance numbers for the model used in production may vary slightly depending on system updates, 昀椀nal parameters, system prompt, and other factors. 3.1 Safety Evaluations Our safety work for o1 builds on prior learning and leverages the wealth of advancements in language model safety. For example, we use a range of public and internally constructed evaluations to measure o1 on tasks such as propensity to generate disallowed content, performance on tasks relevant to demographic fairness [1, 2], tendency to hallucinate [3], and presence of dangerous capabilities [4, 5]. We also build on external red teaming practices learnt over prior models [6, 7, 8, 9] and take advantage of the increasingly mature ecosystem for red teaming. Additionally, o1 inherits our earlier safety mitigations of training in refusal behavior for harmful requests and using moderation models for the most egregious content [10, 11]. 2
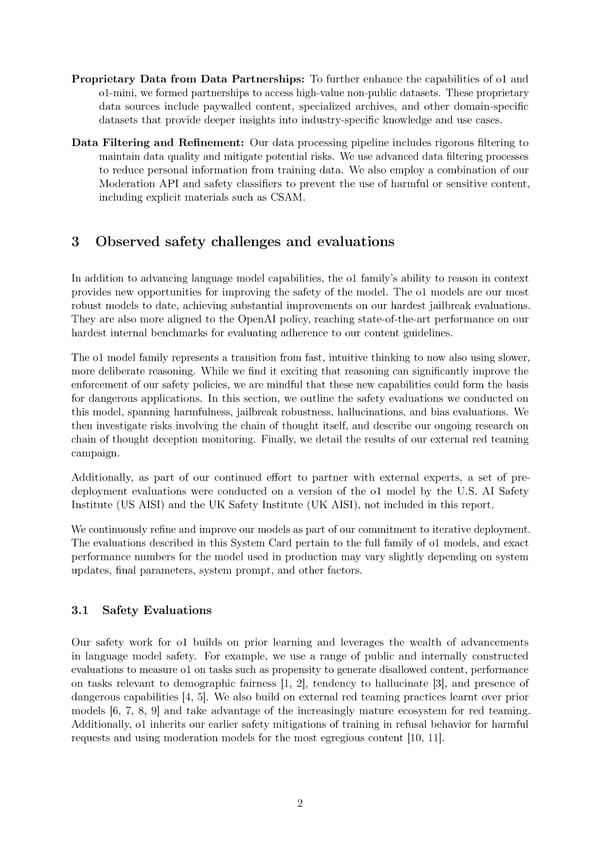