OpenAI o1
This report outlines the safety work carried out prior to releasing OpenAI o1 and o1-mini, including external red teaming and frontier risk evaluations according to our Preparedness Framework.
OpenAI o1 System Card OpenAI December 5, 2024 1 Introduction The o1 model series is trained with large-scale reinforcement learning to reason using chain of thought. These advanced reasoning capabilities provide new avenues for improving the safety and robustness of our models. In particular, our models can reason about our safety policies in context when responding to potentially unsafe prompts. This leads to state-of-the-art performance on certain benchmarks for risks such as generating illicit advice, choosing stereotyped responses, and succumbing to known jailbreaks. Training models to incorporate a chain of thought before answering has the potential to unlock substantial bene昀椀ts, while also increasing potential risks that stem from heightened intelligence. Our results underscore the need for building robust alignment methods, extensively stress-testing their e昀케cacy, and maintaining meticulous risk management protocols. This report outlines the safety work carried out for the OpenAI o1 and OpenAI o1-mini models, including safety evaluations, external red teaming, and Preparedness Framework evaluations. 2 Model data and training The o1 large language model family is trained with reinforcement learning to perform complex reasoning. o1 thinks before it answers4it can produce a long chain of thought before responding to the user. OpenAI o1 is the next model in this series (previously OpenAI o1-preview), while OpenAI o1-mini is a faster version of this model that is particularly e昀昀ective at coding. Through training, the models learn to re昀椀ne their thinking process, try di昀昀erent strategies, and recognize their mistakes. Reasoning allows o1 models to follow speci昀椀c guidelines and model policies we’ve set, helping them act in line with our safety expectations. This means they are better at providing helpful answers and resisting attempts to bypass safety rules, to avoid producing unsafe or inappropriate content. The two models were pre-trained on diverse datasets, including a mix of publicly available data, proprietary data accessed through partnerships, and custom datasets developed in-house, which collectively contribute to the models’ robust reasoning and conversational capabilities. Select Public Data: Both models were trained on a variety of publicly available datasets, including web data and open-source datasets. Key components include reasoning data and scienti昀椀c literature. This ensures that the models are well-versed in both general knowledge and technical topics, enhancing their ability to perform complex reasoning tasks. 1
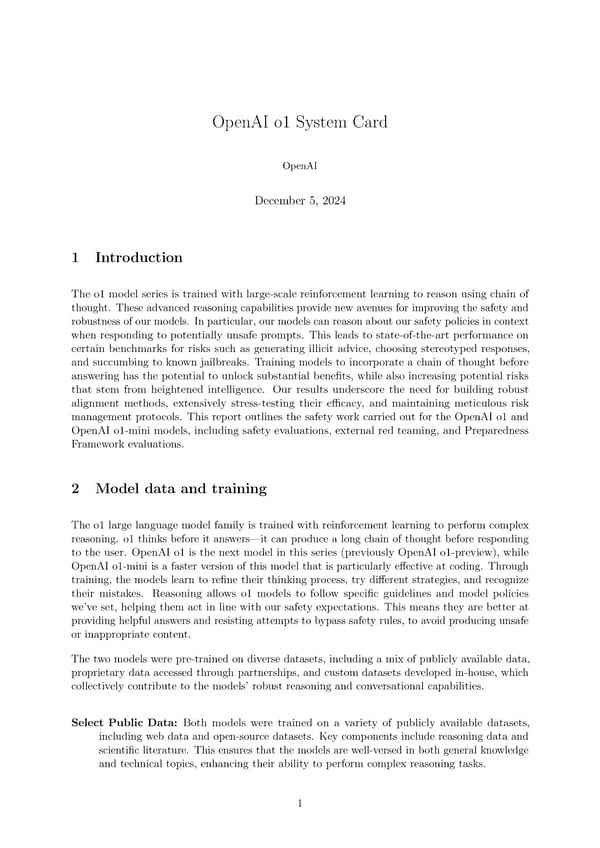