Table 17: MMLU Language (0-shot) Language o1 o1-preview GPT-4o o1-mini GPT-4o-mini Arabic 0.8900 0.8821 0.8155 0.7945 0.7089 Bengali 0.8734 0.8622 0.8007 0.7725 0.6577 Chinese (Simpli昀椀ed) 0.8892 0.8800 0.8335 0.8180 0.7305 English (not translated) 0.9230 0.9080 0.8870 0.8520 0.8200 French 0.8932 0.8861 0.8437 0.8212 0.7659 German 0.8904 0.8573 0.8292 0.8122 0.7431 Hindi 0.8833 0.8782 0.8061 0.7887 0.6916 Indonesian 0.8861 0.8821 0.8344 0.8174 0.7452 Italian 0.8970 0.8872 0.8435 0.8222 0.7640 Japanese 0.8887 0.8788 0.8287 0.8129 0.7255 Korean 0.8824 0.8815 0.8262 0.8020 0.7203 Portuguese (Brazil) 0.8952 0.8859 0.8427 0.8243 0.7677 Spanish 0.8992 0.8893 0.8493 0.8303 0.7737 Swahili 0.8540 0.8479 0.7708 0.7015 0.6191 Yoruba 0.7538 0.7373 0.6195 0.5807 0.4583 6 Conclusion OpenAI o1 performs chain-of-thought reasoning in context, which leads to strong performance across both capabilities and safety benchmarks. These increased capabilities come with sig- ni昀椀cantly improved performance on safety benchmarks, but also increase certain types of risk. Speci昀椀cally, through both internal evaluations and collaborations with external red teamers, we have identi昀椀ed our pre-mitigation models as medium risk in persuasion and CBRN within the OpenAI Preparedness Framework. Overall, o1 has been classi昀椀ed as medium risk in the Preparedness Framework, and we have incorporated commensurate safeguards and safety mitigations to prepare for this new model family. Our deployment of these models re昀氀ects our belief that iterative real-world deployment is the most e昀昀ective way to bring everyone who is a昀昀ected by this technology into the AI safety conversation. 40
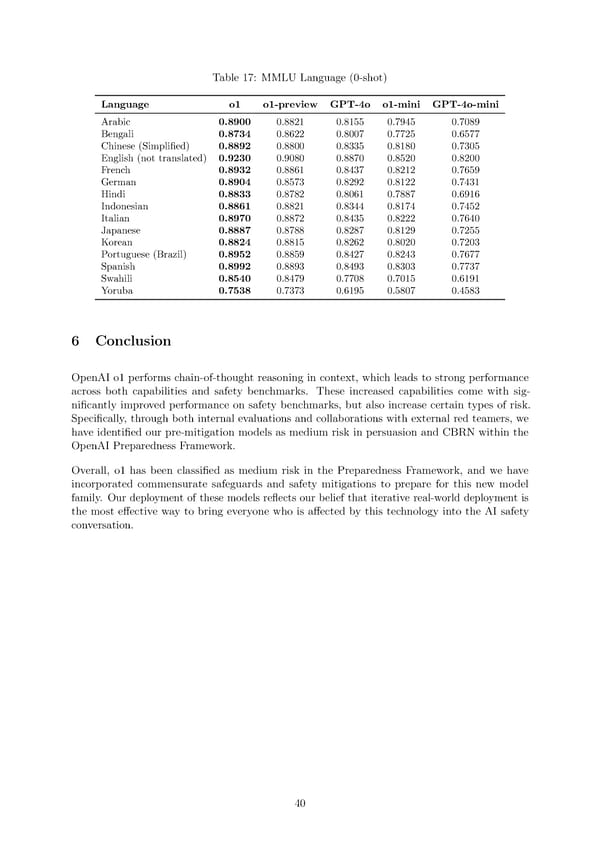