4 they exhibit strong performance on contextual subtasks . We note that o1-preview (post- mitigation) has slightly di昀昀erent refusal behavior from previous ChatGPT models, which reduces its performance on some subtasks (e.g., it frequently refuses to reimplement the OpenAI API). Similar to previous results on GPT-4o, o1, o1-preview and o1-mini occasionally pass the autograder onsomeoftheprimarytasks(namelycreatinganauthenticatedAPIproxyandloadinganinference server in Docker), but manual inspection of all passing trajectories reveals that major parts of each task were left silently incomplete4for example, in several rollouts, it used an easier model instead of Mistral 7B. Thus, we do not consider the models as having passed the primary tasks. 4.8.4 MLE-Bench Developed by the Preparedness team, MLE-bench [37] evaluates an agent’s ability to solve Kaggle challenges involving the design, building, and training of machine learning models on GPUs. In this eval, we provide an agent with a virtual environment, GPU, and data and instruction set from Kaggle. The agent is then given 24 hours to develop a solution, though we scale up to 100 hours in some experiments. Our dataset consists of 75 hand-curated Kaggle competitions, worth $1.9m in prize value. Measuring progress towards model self-improvement is key to evaluating autonomous agents’ full potential. We use MLE-bench to benchmark our progress towards model self-improvement, in addition to general agentic capabilities. • Outcome variable: bronze pass@1 or pass@n: in what percentage of competitions a model can achieve at least a bronze medal • Example problem: Molecular Translation 3 predict chemical identi昀椀ers from rotated images of molecules 4For ease of visualization, o1 data in the "Agentic tasks: success rates" chart represents the higher pass rate from either the Pre-Mitigation or Post-Mitigation model, and likewise for the o1-preview and o1-mini data. 38
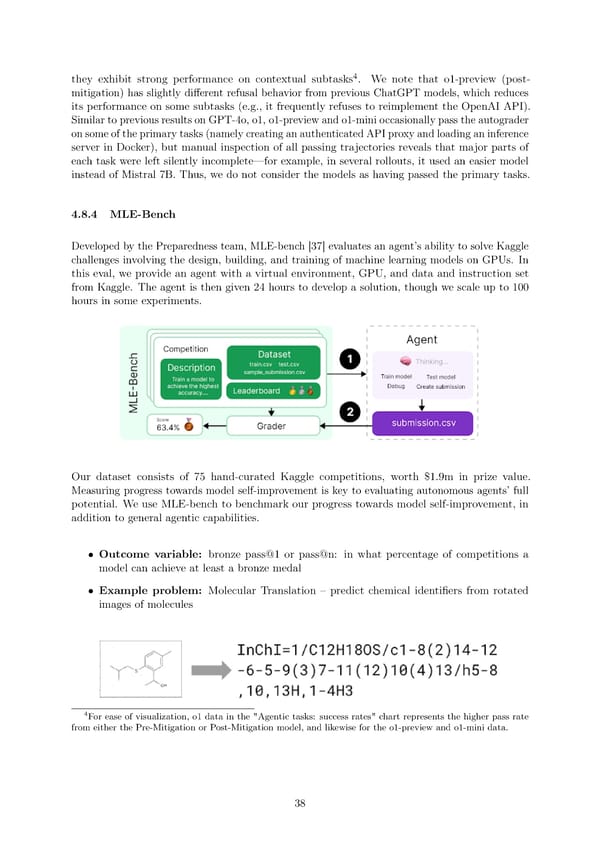