GPT-4o is cheaper and thus more widely available than its predecessor GPT-4T, and the addition of audio inputs and outputs presents new modes of interaction in health settings. To better characterize the clinical knowledge of GPT-4o, we ran 22 text-based evaluations based on 11 datasets, shown in 7. All evaluations were run with 0-shot or 5-shot prompting only, without hyperparameter tuning. We observe that GPT-4o performance improves over the 昀椀nal GPT-4T model for 21/22 evaluations, often by a substantial margin. For example, for the popular MedQA USMLE 4 options dataset, 0-shot accuracy improves from 78.2% to 89.4%. This exceeds the performance of existing specialized medical models using few-shot prompting [43, 42], e.g., 84.0% for Med-Gemini-L 1.0 and 79.7% for Med-PaLM 2. Note that we do not apply sophisticated prompting and task-speci昀椀c training to improve results on these benchmarks [40, 43]. GPT-4T (May GPT-4o 2024) MedQAUSMLE4Options (0-shot) 0.78 0.89 MedQAUSMLE4Options (5-shot) 0.81 0.89 MedQAUSMLE5Options (0-shot) 0.75 0.86 MedQAUSMLE5Options (5-shot) 0.78 0.87 MedQATaiwan (0-shot) 0.82 0.91 MedQATaiwan (5-shot) 0.86 0.91 MedQAMainland China (0-shot) 0.72 0.84 MedQAMainland China (5-shot) 0.78 0.86 MMLUClinical Knowledge (0-shot) 0.85 0.92 MMLUClinical Knowledge (5-shot) 0.87 0.92 MMLUMedical Genetics (0-shot) 0.93 0.96 MMLUMedical Genetics (5-shot) 0.95 0.95 MMLUAnatomy(0-shot) 0.79 0.89 MMLUAnatomy(5-shot) 0.85 0.89 MMLUProfessional Medicine (0-shot) 0.92 0.94 MMLUProfessional Medicine (5-shot) 0.92 0.94 MMLUCollege Biology (0-shot) 0.93 0.95 MMLUCollege Biology (5-shot) 0.95 0.95 MMLUCollege Medicine (0-shot) 0.74 0.84 MMLUCollege Medicine (5-shot) 0.80 0.89 MedMCQADev(0-shot) 0.70 0.77 MedMCQADev(5-shot) 0.72 0.79 Table 7: Comparison of GPT-4T (May 2024) and GPT-4o on various medical and clinical knowledge tasks. Limitations While text-based evaluations appear promising, additional future work is needed to test whether text-audio transfer, which occurred for refusal behavior, extends to these evaluations. These evaluations measure only the clinical knowledge of these models, and do not measure their utility in real-world work昀氀ows. Many of these evaluations are increasingly saturated, and we believe that more realistic evaluations will be important for assessing the future capabilities of omni models in health settings. 21
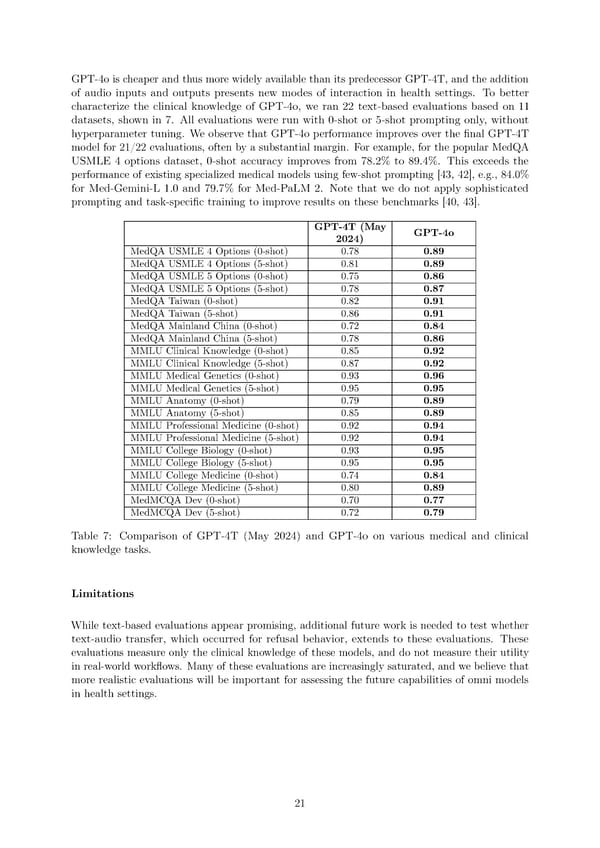