4 System Safety 4.1 Usage Policies and Monitoring OpenAI disallows the use of our models and tools for certain activities and content, as outlined in our usage policies. These policies are designed to prohibit the use of our models and tools in ways that cause individual or societal harm. We update these policies in response to new risks and new information on how our models are being used. Access to and use of our models are also subject to OpenAIs Terms of Use. We use a mix of reviewers and automated systems to identify and enforce against misuse of our models. Our automated systems include a suite of machine learning and rule-based classifier detections that identify content that might violate our policies. When a user repeatedly prompts our models with policy-violating content, we take actions such as issuing a warning, temporarily suspending, or in severe cases, banning the user. Our reviewers ensure that our classifiers are correctly blocking violative content and understand how users are interacting with our systems. These systems also create signals that we use to mitigate abusive and inauthentic behavior on our platform. We investigate anomalies in API traffic to learn about new types of abuse and to improve our policies and enforcement. 4.2 Content Classifier Development Moderation classifiers play a key role in our monitoring and enforcement pipeline. We are constantly developing and improving these classifiers. Several of our moderation classifiers are accessible to developers via our Moderation API endpoint, which enables developers to filter out harmful content while integrating language models into their products. Wehave also experimented with building classifiers using the GPT-4 model itself, and have been studying the effectiveness of various approaches to doing so.31 Given GPT-4’s heightened ability to follow instructions in natural language, the model was able to accelerate the development of moderation classifiers and augment safety workflows. This was done in two ways: 1. The model helped speed up development of robust, unambiguous taxonomies needed for content classification (i.e. content policies). This included classifying test sets when prompted with a taxonomy, enabling an assessment of prompts that it labeled incorrectly by identifying gaps in the taxonomy that led to the incorrect label. 2. The model helped facilitate the labeling of training data that was fed into classifier training; the model demonstrated high performance on few-shot classification, which helped to bootstrap the creation of labeled data for human review. Harnessing GPT-4 in this manner enables us to build classifiers for new content areas faster than before.[101] We continue to provide oversight for quality control and for input on edge cases.32 We note that further and ongoing testing is required to ensure that classifiers dont exacerbate inequalities or biases in content moderation decisions. Finally, as we discuss above in the Overreliance section product-level features and documentation such as warnings and user education documents are essential to responsible uptake of increasingly powerful language models like GPT-4. 31We will be sharing more about this work in a forthcoming publication. 32Content classifiers cannot fix all issues related with content harms and can themselves be a source of harms by potentially exacerbating bias in content moderation decisions.[105] 66
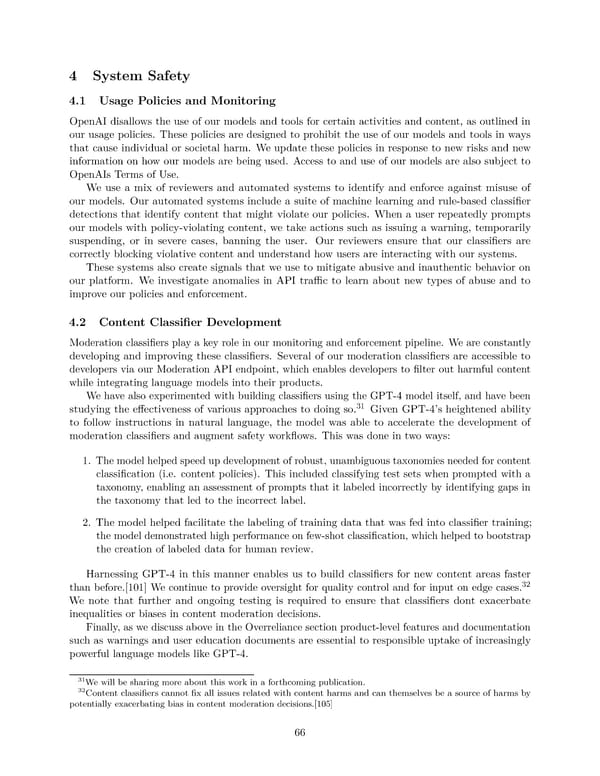