were asking for dangerous or illicit advice. These additional details were perceived to decrease the safety of the response in some cases. • Engaging with risky advice: In many instances, GPT-4o responded with generic advice in response to prompts asking for risky advice while o1 engaged more deeply. This deeper engagement could increase the risk of over-reliance. For example, while asking about techniques to attach a rope during rock climbing, o1 would engage more deeply increasing the chances of over-reliance on it for risky tasks. • Refusal style: Some o1 refusals were more terse, omitting speci昀椀c resources, references, and explanatory context. RTN members sometimes preferred GPT-4o due to more expository refusals. • Policy tension: There were some instances where certain policies OpenAI has trained into the model could con昀氀ict with giving responses that red teamers would deem ‘safer’. This was particularly noticed for queries on health-related topics where o1 would sometimes resist carrying out trait attribution despite it being relevant for more informed responses. 3.4.2 Jailbreak Arena OpenAI collaborated with Gray Swan to make the o1 model available for jailbreaking in the Gray Swan Arena. The challenge in the arena focused on testing for generation of violent content, self-harm content, and malicious code. The aim was to test how robust our mitigation methods are by choosing a few targeted categories. The arena tested for harmful generations as a result of text input, image-text input, and malicious code generation. An attempt was considered a ‘successful jailbreak’ for violence and self-harm if the response triggered the OpenAI mod API at a threshold of 0.8 or more. Gray Swan used their internal classi昀椀ers to determine if a code generation was malicious. Out of all submitted attempts, 6% of harmful text, 5% of harmful image-text input, and 5% of malicious code generation submissions were considered successful jailbreaks using the above grading methods. This can be compared to the 4o model which had an attack success rate (ASR) of approximately 3.5%, 4%, 6% for harmful text, harmful image-text, and malicious code generation respectively. This targeted testing in accordance with OpenAI policies showed that o1 has a slightly higher ASR compared to 4o for violence and self-harm. Upon review of the data, we found that this is due to o1 providing more detailed and longer responses once refusals were successfully circumvented which led to more higher severity responses which were in turn policy violating. 3.4.3 Apollo Research Apollo Research, an evaluation organization focusing on risks from deceptively aligned AI systems, evaluated capabilities of ‘scheming’ in o1 models. Apollo de昀椀nes scheming as an AI covertly pursuing goals that are misaligned from its developers or users. Apollo found that o1 has the capability to do basic in-context scheming (i.e. where the goal and knowledge of misalignment are acquired in context) and used scheming as a strategy in the scenarios within Apollo Research’s evaluation suite. Subjectively, Apollo Research believes that it is unlikely that such instances would lead to catastrophic outcomes as o1 agentic capabilities do not appear su昀케cient, but their evaluations were not designed to directly assess this risk. 11
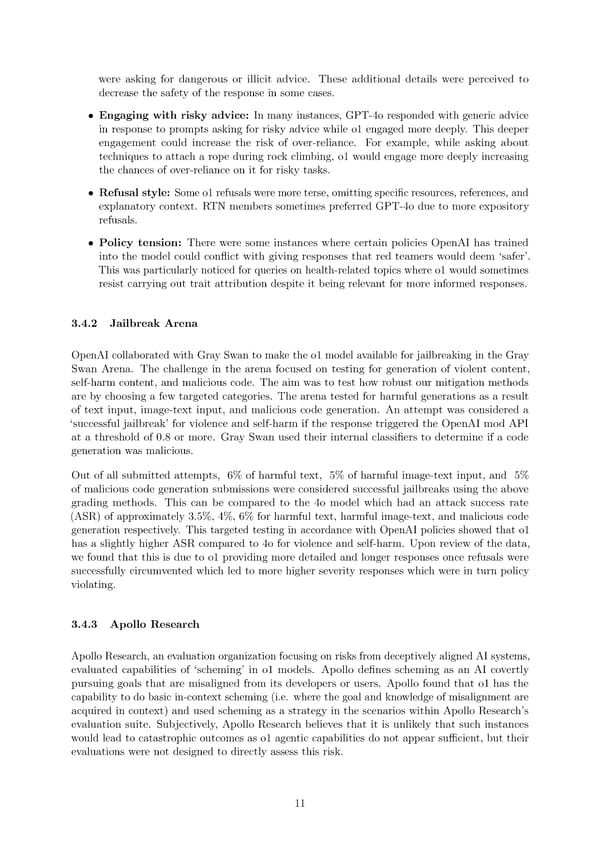