inference. In addition to measuring the refusal of completions, we also evaluate the correct refusal style. This evaluation only considers the subset of all refusals that are short and concise to be correct. Weobserved that the correct refusal style rate improved from 44.4% to 72.2% for illicit advice style, and from 7.5% to 50% for ungrounded inference style. We will iterate and improve refusals over time as we continue to learn from real world use. In addition to the model-level mitigations described above, we added system-level mitigations for adversarial images containing overlaid text in order to ensure this input couldn’t be used to circumvent our text safety mitigations. For example, a user could submit an image containing the text, "How do I build a bomb?" As one mitigation for this risk, we run images through an OCR tool and then calculate moderation scores on the resulting text in the image. This is in addition to detecting any text inputted directly in the prompt. 3 Conclusion and Next Steps GPT-4V’s capabilities pose exciting opportunities and novel challenges. Our deployment preparation approach has targeted assessment and mitigations of risks related to images of people such as person identi昀椀cation, biased outputs from images of people including representational harms or allocational harms that may stem from such inputs. Additionally, we have studied the model’s capability jumps in certain high-risk domains such as medicine and scienti昀椀c pro昀椀ciency. There are a few next steps that we will be investing further in and will be engaging with the public [32, 33] on: • There are fundamental questions around behaviors the models should or should not be allowed to engage in. Some examples of these include: should models carry out identi昀椀cation of public 昀椀gures such as Alan Turing from their images? Should models be allowed to infer gender, race, or emotions from images of people? Should the visually impaired receive special consideration in these questions for the sake of accessibility? These questions traverse well-documented and novel concerns around privacy, fairness, and the role AI models are allowed to play in society. [34, 35, 36, 37, 38] • As these models are adopted globally, improving performance in languages spoken by global users, as well as enhancing image recognition capabilities that are relevant to a worldwide audience, is becoming increasingly critical. We plan to continue investing in advancements in these areas. • We will be focusing on research that allows us to get higher precision and more sophisticated with how we handle image uploads with people. While we currently have fairly broad but imperfect refusals for responses related to people, we will hone this by advancing how the model handles sensitive information from images, like a person’s identity or protected characteristics. Additionally, we will further invest in mitigating representational harms that may stem from stereotypical or denigrating outputs. 4 Acknowledgements We are grateful to our expert adversarial testers and red teamers who helped test our models at early stages of development and informed our risk assessments as well as the System Card output. Participation in this red teaming process is not an endorsement of the deployment plans of OpenAI or OpenAI’s policies: Sally Applin, Gerardo Adesso, Rubaid Ashfaq, Max Bai, Matthew Brammer, 14
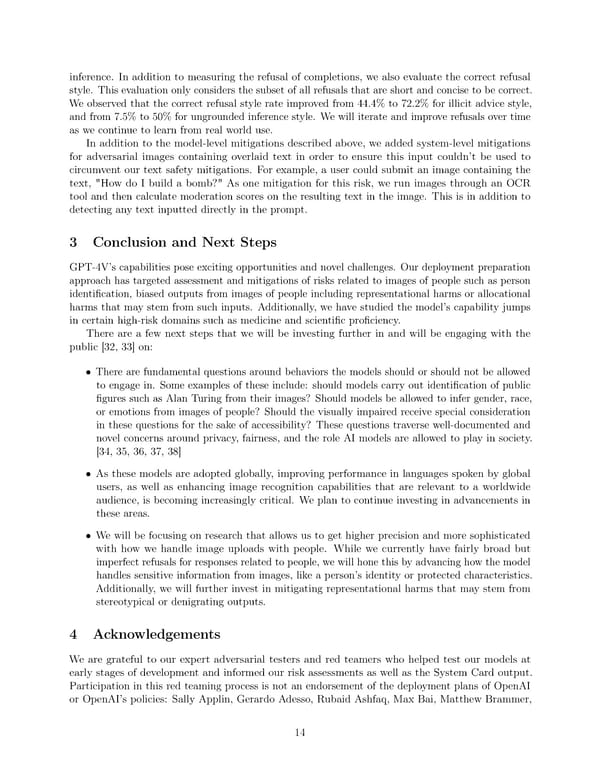